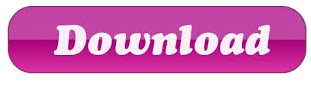

In the statistical significance post, the scientist went in with a well-motivated hypothesis which she put to the test in an experiment. It is one of the most common ways in which data analysis is misused to generate statistically significant results where none exists, and is one which everyone reporting on science should remain vigilant against. This piece introduces one such technique known as ‘p-hacking’. To avoid reporting spurious results as fact and giving air to bad science, journalists must be able to recognise when such methods may be in use.
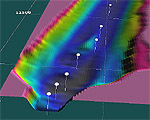
However, there are ways in which statistical techniques can be misused and abused to show effects which are not really there. Most scientists are careful and scrupulous in how they collect data and carry out statistical tests. It is a misuse of data analysis to find patterns in data that can be presented as statistically significant when in fact there is no real underlying effect. This is a technique known colloquially as ‘p-hacking’. In this post, we will look at a way in which this process can be abused to create misleading results. In our explainer on statistical significance and statistical testing, we introduced how you go about testing a hypothesis, and what can be legitimately inferred from the results of a statistical test.
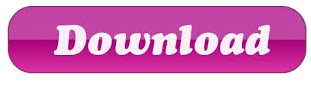